In this latest Target Tech Bytes, we’re covering the tech trend that has recently taken the world by storm, Generative Artificial Intelligence (AI), also known as GenAI. The hype around this technology is louder than ever, and to the uninitiated, it could be overwhelming. It's flooding headlines and social media, making it hard to ignore, so let’s explore what it’s all about and how it’s transforming content creation.
Before we dig deeper, let’s look at Gartner’s definition of Generative AI:
Generative AI refers to AI techniques that learn a representation of artifacts from data, and use it to generate brand-new, unique artifacts that resemble but don’t repeat the original data. These artifacts can serve benign or nefarious purposes. Generative AI can produce totally novel content (including text, images, video, audio, structures), computer code, synthetic data, workflows and models of physical objects. Generative AI also can be used in art, drug discovery or material design.
So, what is it?
In reality, it’s no longer an innovative concept; it can speak your language, and there are now real-world examples of Generative AI that can not only understand context, but even understand humour!
Generative AI is a subset of AI technology that uses Natural Language Processing (NLP) and machine learning to produce various types of content, including text, imagery, and audio, which mimics human-generated content.
Unlike traditional AI algorithms that recognise and classify data to make predictions from a training data set, Generative AI uses machine learning and vast amounts of existing training data to identify patterns and generate new content through its learning.
The content can range from writing essays or new songs to generating a convincing image of a human that doesn’t exist in the real world. The more detailed and diverse the training data the model reviews, the better the quality of output that it produces.
A brief history
Artificial intelligence has a long and often controversial history; the concept of machine thinking has been talked about for centuries. AI as we know it started to take shape in the 1950s due to the work of Alan Turing and his introduction of the ‘Turing Test’.
Generative AI has been an active research topic since the 1960s, with Joseph Weizenbaum developing the first Natural Language Processing (NLP) chatbot called ELIZA.
In the 1980s, research into using neural network algorithms to generate new content became popular.
Advancement in Generative AI leapt forward in 2014 with the introduction of Generative Adversarial Networks (GANs) by Ian Goodfellow. His technique uses two neural networks competing against each other using deep learning methods, making them more accurate in their responses. It became one of the most popular techniques for Generative AI. GANs have gained popularity in image creation and modification.
In more recent years, developments of large-scale neural networks and the accessibility of large datasets have paved the way for Generative AI technology to flourish. As the years pass, we continuously push the boundaries of what is possible.
Examples of Generative AI applications
Artificial intelligence has been on Gartner’s technology Hype Cycle since 2020. However, Generative AI only hit mainstream public headlines in November 2022 with the launch of ChatGPT by Open AI. Almost overnight, the Generative AI chatbot went viral, and within just a few days, it attracted over one million users.
A recent poll by Gartner found that 55% of organisations reported increasing investment in Generative AI since it surged into the public domain in 2022.
Microsoft launched Bing Chat in February 2023. Integrated into its search engine, Bing Chat, now known as Microsoft Copilot, it allows users to interact with an AI-powered, conversational chatbot that can assist with various tasks and queries. Microsoft Copilot is based on OpenAI's ChatGPT-4 model, with some modifications and enhancements.
In March 2023, Google launched its version of an AI powered chatbot called Bard after previously making headlines due to it giving an incorrect answer during a demo.
Natwest have recently hit the news to confirm a collaboration with IBM to upgrade their existing chatbot Cora. Their new digital assistant, Cora+, will use Generative AI to enhance customer experience through the chatbot's ability to answer customer queries in a more 'natural and conversational-like' way.
Pros and cons
The rise in public interest in this innovative technology and the potential it has continues to grow, let’s look at some pros and cons of Generative AI:
Pros
- Creative potential - Producing original content, Generative AI empowers individuals and organisations with vast content generation possibilities
- Time efficiency - The time and effort saved by using Generative AI is significant. It can generate large volumes of content in next to no time, reducing the amount of manual work required
- Customisation - The technology can interpret human language, which allows it to generate relevant responses in the correct context to the human prompt
- Problem solving - Generative AI can help with complex problem solving tasks by creating novel and diverse solutions that are tailored to the specific needs and constraints of the problem domain
- Various applications - Generative AI can be applied to many different tasks in a variety of sectors, such as chatbots for Customer Services, content creation for Marketing, language translation or code writing.
Cons
- Accuracy - Generative AI applications may produce inaccurate, biased or false outputs depending on the training data it's reviewed to produce new content. Care would need to be taken before relying on any content produced
- Sustainability and carbon footprint - The energy consumption from Generative AI is significant
- Cybersecurity and privacy risks - Harmful requests that can mimic senior management and cyber security attacks on businesses pose a big risk when using GenAI
- Deepfakes - Malicious deep fakes, where the manipulation of facial appearance to create audio and visual content to deceive is becoming more common
- Job displacement - Concerns are growing that Generative AI could displace many creative jobs.*
Who’s using Generative AI
Thanks to advances in technology such as ChatGPT, GenAI is becoming more accessible to many audiences. Some use cases for GenAI include:
- Chatbots for customer services and technical support - Many industries have already branched out into this area including banking, retail and many more
- Advertisements or social media content for marketing - It could help produce draft content in the tone and style required, saving time and resource
- Software coding - The technology could help improve or rewrite software code to enhance legacy systems
- Personalised product suggestions - Generating customised recommendations for customer products.
A survey conducted by Mckinsey’s in 2022 shows that investment in AI and its adoption has more than doubled over the last five years, since 2017.
Generative AI in Financial Services
Generative AI has the power to create personalised customer experiences by generating relevant and engaging content, such as conversational journeys, product recommendations, and feedback.
It could be used to enhance fraud detection capabilities by generating realistic synthetic data, such as voice, face, and text, to train and test models.
Generative AI also has potential in risk management. It could be used to generate scenarios, simulations, and forecasts, such as stress testing, market analysis, and portfolio optimisation.
Large Language Models and Generative AI can also open opportunities for knowledge management. Knowledge is key within any business, but it’s often stored across the organisation in many different formats including processes, policies, online chats and in some cases on individuals’ desktops! This can make it difficult to leverage crucial knowledge in the most effective way, emerging technologies can step in to capture and distribute this knowledge.
There are three primary approaches to training a generative model with business knowledge, let’s take a brief look:
- Training a Large Language Model from scratch - The approach to creating and training a business specific Large Language Model is no easy feat. It requires a vast amount of high-quality company data and access to considerable computer power, which many businesses simply don’t have
- Modify an existing Large Language Model - Fine-tuning an existing Large Language model involves making some adjustments to the base model, which is already trained on general knowledge and then train with new business specific content. It typically requires substantially less data compared to the approach above
- Prompt-tuning an existing Large Language Model - Keeping the original model unchanged, this model is trained through new business specific prompts in the context window. Following prompt-tuning, the model can answer questions relating to the business knowledge.
How could Generative AI benefit your business?
Generative AI is only as good as the training data, inputs and questioning that it relies on to create accurate outputs. If the training data is biased, incomplete, inaccurate, or outdated, then the Generative AI output will reflect those flaws and may not be reliable or useful. Similarly, if the inputs and questioning are vague, ambiguous, irrelevant, or inappropriate, then the Generative AI output won’t meet the expectations or needs of the user. It’s important to ensure that the training data inputs and questioning are of high quality and relevance when using Generative AI.
To improve Generative AI questioning, companies should:
- Describe the goal and scope of the question clearly and precisely
- Use natural language and avoid jargon, acronyms, or technical terms
- Provide context and background information that can help the AI model generate more relevant and accurate outputs
- Use open-ended questions that invite multiple possible answers, rather than closed-ended questions that create a single answer.
Moving forward, Generative AI has the potential to transform the way businesses operate, it could help write code, develop new products, or even redesign and automate processes. It could take customer services to the next level, turbocharging experiences and anticipating customers' needs.
Generative AI is a game-changer, but not a magic bullet. It can help you create amazing content, products, and solutions, but it also comes with costs and challenges. Before you adopt it, you need to consider how it will affect your business. Ask yourself if the benefits outweigh the cost to implement and maintain, and if being ahead in the digital transformation race can give you an edge over the competition. If yes, then now's the time to act!
* It's anticipated that more jobs will become available in Large Language Model prompting and Generative AI business implementation.
Sources
https://bernardmarr.com/a-simple-guide-to-the-history-of-generative-ai/
https://www.gartner.com/en/topics/generative-ai
https://www.techtarget.com/searchenterpriseai/definition/generative-AI
https://writer.com/blog/generative-ai-defined-hype-vs-reality/
https://hbr.org/2023/07/how-to-train-generative-ai-using-your-companys-data
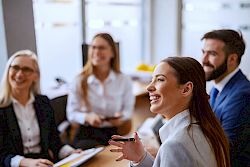